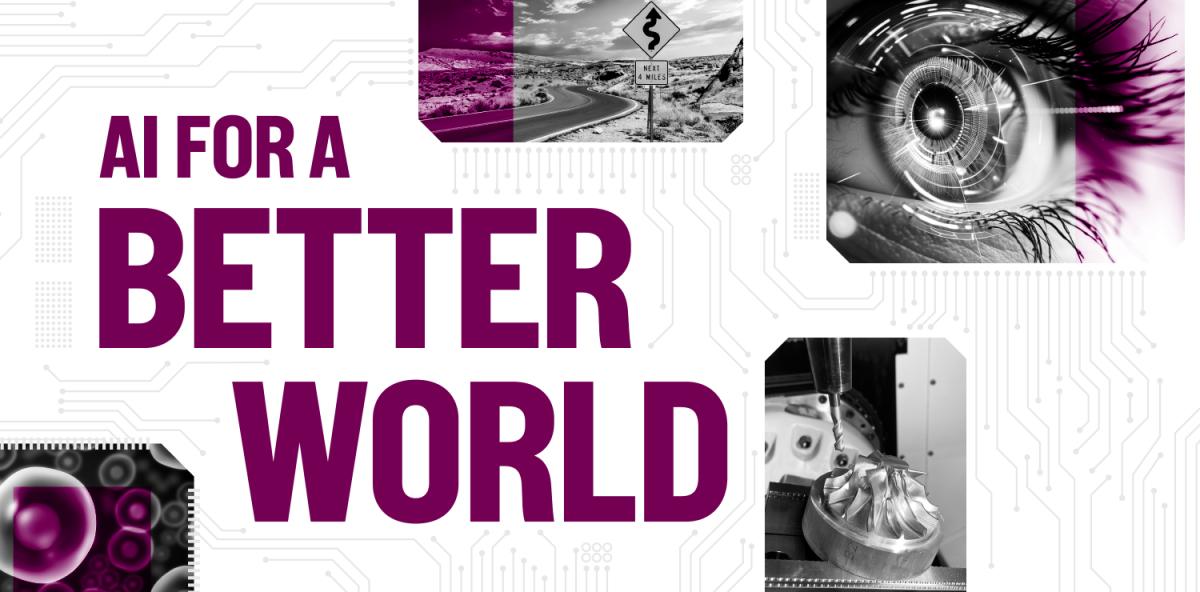
(text and background only visible when logged in)
Georgia Tech engineers are refining AI tools and deploying them to help individuals, cities, and everything in between.
(text and background only visible when logged in)
Artificial intelligence and machine learning techniques are infused across the College of Engineering’s education and research.
From safer roads to new fuel cell technology, semiconductor designs to restoring bodily functions, Georgia Tech engineers are capitalizing on the power of AI to quickly make predictions or see danger ahead.
Here are a few ways we are using AI to create a better future.
(text and background only visible when logged in)
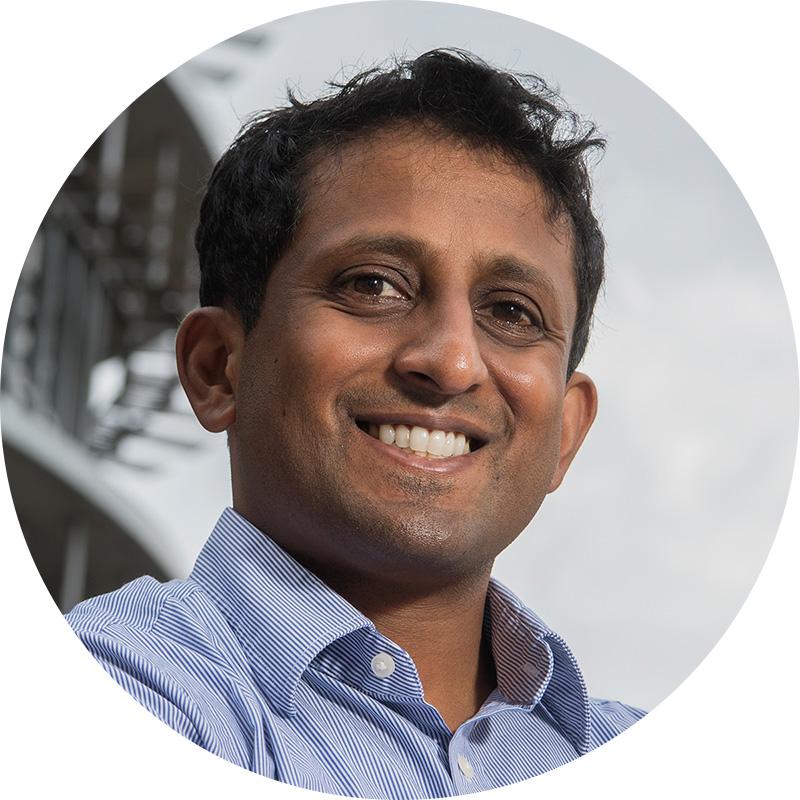
Chethan Pandarinath (Photo: Jack Krause)
Reconnecting Body and Brain
A partnership between biomedical engineers and Emory neurologists is using AI to help patients paralyzed from strokes, spinal cord injuries, or other conditions move again. Led by Chethan Pandarinath, the project aims to create brain-machine interfaces that can decode in just milliseconds, and with unprecedented accuracy, what the brain is telling the body to do. In essence, they’re trying to reconnect the brain and body for these patients.
Using a machine learning concept called “unsupervised” or “self-supervised” learning, the team is taking a new approach to understanding brain signals. Rather than starting with a movement and trying to map it to specific brain activity, Pandarinath’s algorithms start with the brain data.
“We don’t worry about what the person was trying to do. If we did, we’d miss a lot of the structure of the activity. If we can just understand the data better first, without biasing it by what we think the pattern meant, it ends up leading to better what we call ‘decoding,’” he said.
The goal is allowing these AI-powered brain-machine interfaces to work for any patient essentially out of the box — no significant calibration needed. The researchers have been working on a clinical trial focused on patients with amyotrophic lateral sclerosis (more commonly known as ALS or Lou Gehrig’s disease).
(text and background only visible when logged in)
The Route to Safer Roads
Curves account for only about 5% of roadway miles in the United States, yet those sections of road are responsible for 25% of all traffic-related deaths. A project led by civil engineer Yi-Chang “James” Tsai is using smartphones and AI to cut into that number, with the potential to save millions of lives.
Relatively simple fixes, like the right signage alerting drivers to curves and suggesting safe speed limits, are known to reduce crashes. But safety assessments are manual, time-consuming endeavors. And they have to be done regularly: Safety conditions change as pavement deteriorates or when weather is bad, and road maintenance or resurfacing can change the curve’s geometry.
Tsai’s solution is to mount a low-cost smartphone in Georgia Department of Transportation (GDOT) vehicles that record video and spatial data from the phone’s onboard gyroscope. Algorithms process the data and flag curves that need attention from traffic engineers. Best of all, the data is collected while GDOT workers go about their daily work without special effort or stops to evaluate dangerous curves.
“Our work saves lives and produces a great positive impact on our community and society,” Tsai said. “The current manual curve safety assessment is labor-intensive, costly, and dangerous to traffic engineers. It typically takes a couple of years to complete the safety assessment on state-maintained roadways in Georgia.”
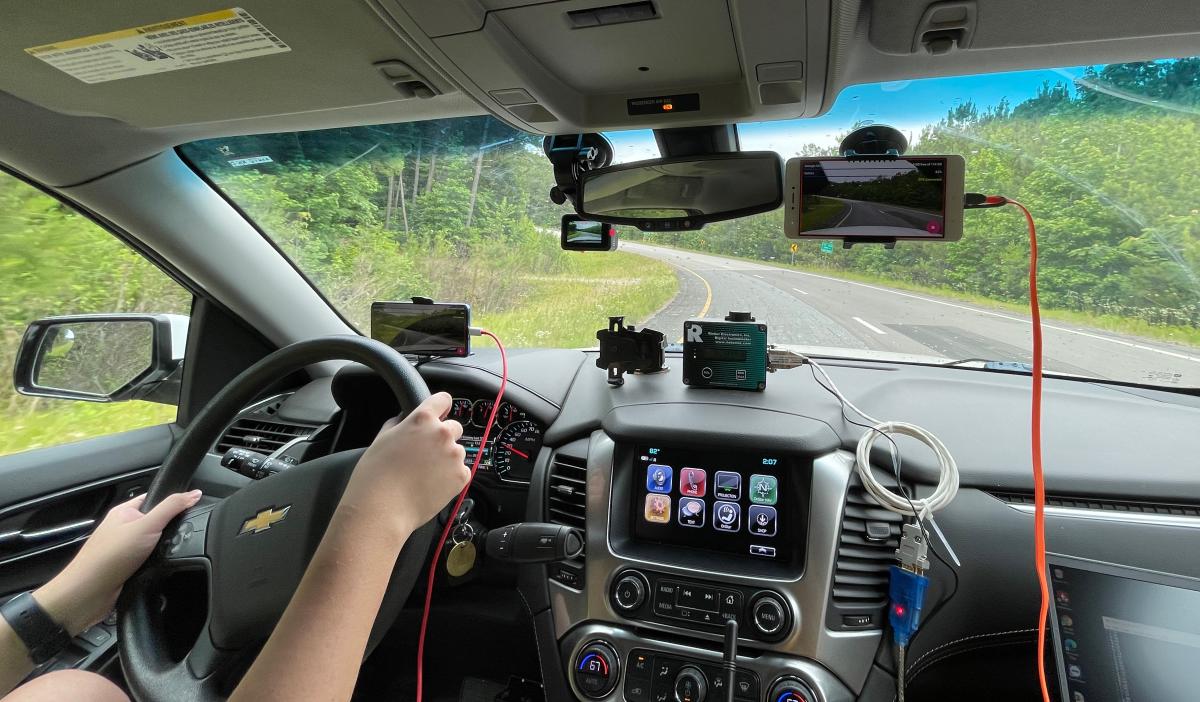
Dash mounted smartphones and AI are helping Georgia’s transportation agency evaluate road curve safety more simply and quickly. (Photo courtesy: James Tsai)
An early version of Tsai’s system already has cataloged Georgia’s 18,000 miles of state-maintained roads, proving its worth to GDOT. Tsai’s team is working to scale up the project, processing data directly on the smartphone and perhaps one day feeding it to Google Maps and other wayfinding apps. Tsai said the idea would be to give drivers real-time alerts when they’re approaching dangerous areas. His vision is to allow Maps users to select a route that’s safest, not just fastest or shortest.
(text and background only visible when logged in)
Speeding Atomic Simulations
Chemical and civil engineers are working together to use the power of AI to supercharge a workhorse approach to modeling chemical interactions and materials properties at the atomic level. The results could help researchers more quickly and accurately make predictions about catalysis, chemical separations, and the mechanical properties of materials.
The researchers are using new machine learning techniques to overcome limitations of an approach called density functional theory (DFT). It’s a powerful tool for calculating materials properties based on the interactions of atoms and electrons. But the computing power required can be enormous because real materials involve billions of atoms interacting over long periods of time.
AJ Medford’s research team in the School of Chemical and Biomolecular Engineering is using the newest machine learning techniques to overcome the limitations while still maintaining the accuracy and reliability of the DFT approach.
“Because of some technical details of DFT, doubling the size of a system means it takes about eight times longer to calculate, so direct calculations of real materials properties become computationally impossible very fast,” Medford said. “Machine learning and AI promise to help overcome this barrier by directly predicting the result of DFT calculations in a way that is much faster and can be more easily scaled up to larger systems.”
Medford is collaborating with Phanish Suryanarayana in the School of Civil and Environmental Engineering (CEE), who developed a DFT simulation package called SPARC. They’re tightly coupling Medford’s machine learning models to that code to produce more reliable acceleration of materials simulations.
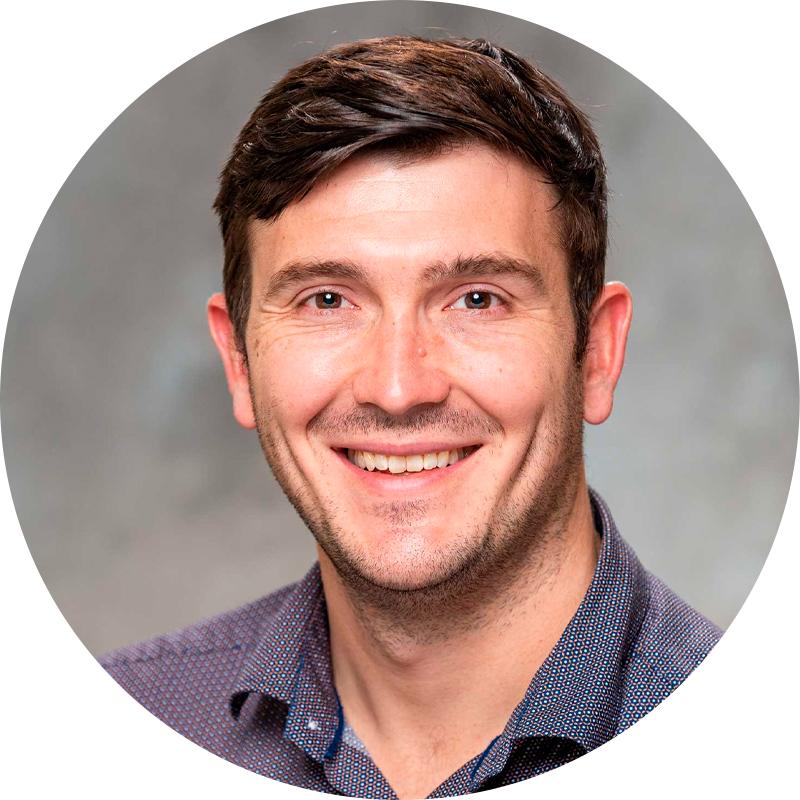
AJ Medford (Photo: Gary Meek)
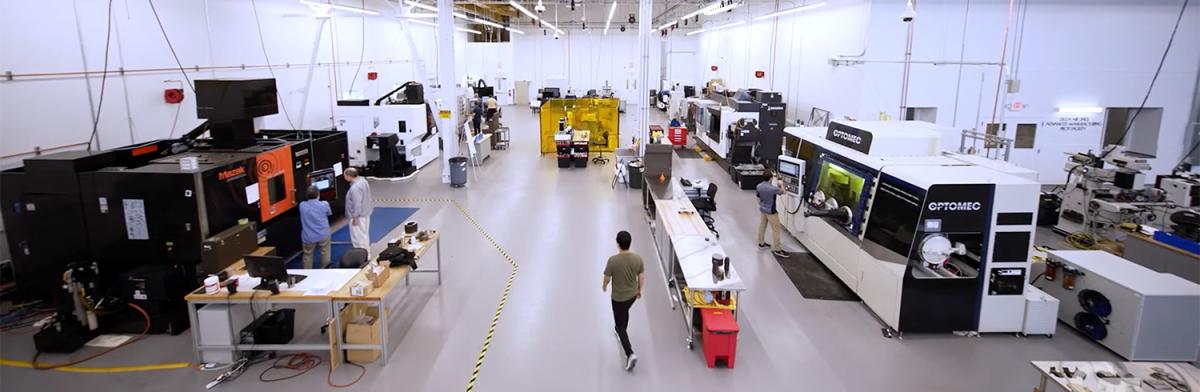
The Advanced Manufacturing Pilot Facility (Courtesy: Georgia Tech Manufacturing Institute)
AI-ing Georgia’s Manufacturing Renaissance
Looking across the 20,000 square feet of Georgia Tech’s Advanced Manufacturing Pilot Facility (AMPF), Aaron Stebner is greeted by a maze of machines. Spread throughout the bright, cavernous space are metal printers with electron beams. A robotic welder. A robotic loader and unloader.
It’s been more than a year and a half since the White House announced a $65 million grant that put Georgia Tech at the forefront of Georgia’s capabilities in artificial intelligence and manufacturing, with AMPF serving as the heart.
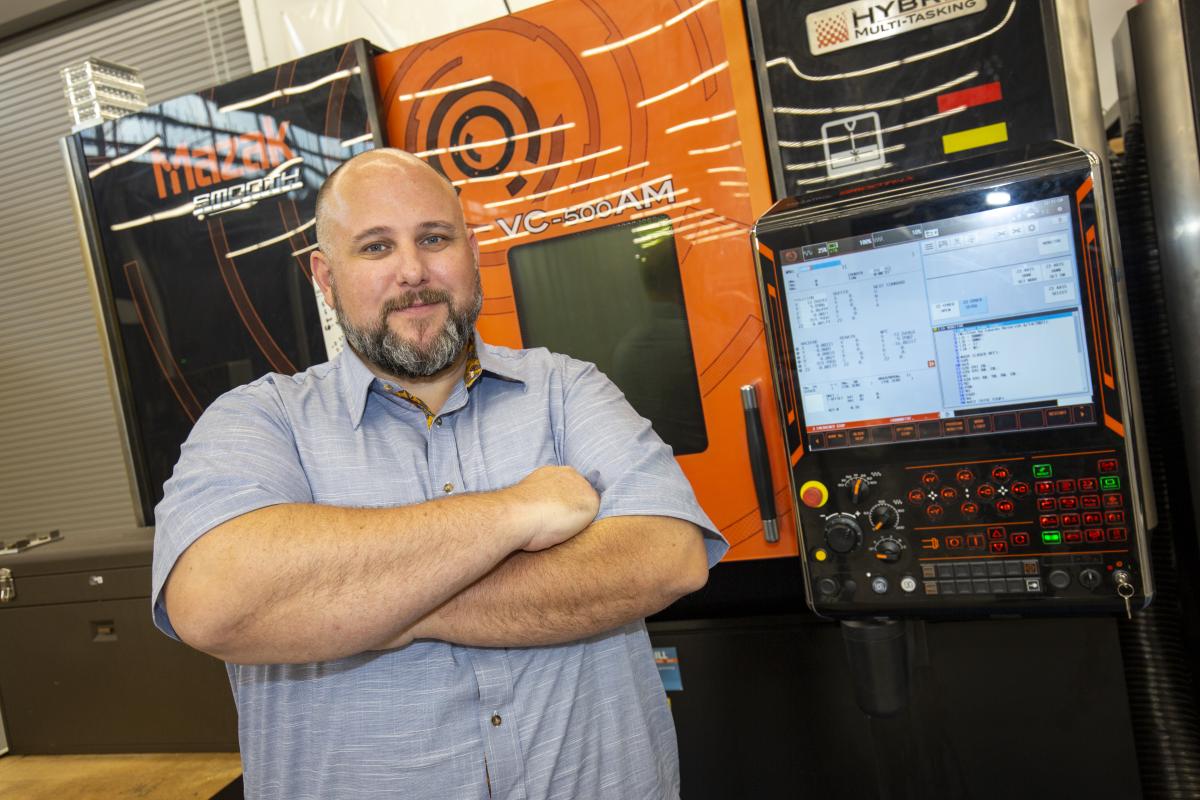
Aaron Stebner (Photo: Candler Hobbs)
“Everything is going gangbusters,” Stebner said recently. “It’s exciting to think about how much we’ve done in the last 18 months.”
The $65 million is bolstering AMPF, a testbed where basic research results are scaled up and translated into implementable technologies, including additive/hybrid manufacturing, composites, and industrial robotics.
Stebner is an associate professor in the George W. Woodruff School of Mechanical Engineering and the School of Materials Science and Engineering. His primary role since that 2022 announcement has been leading the largest of nine projects within the Georgia AI Manufacturing technology corridor grant from the U.S. Department of Commerce’s Economic Development Administration.
Forty or so grad students and five faculty members worked in AMPF when the funding was announced. Now it’s 70 grad students, a dozen faculty, and 50 undergrads, as well as other staff members.
“In addition to more people, we are working with corporate partners on 5G and cloud computing projects,” Stebner said. “It’s busy, and I feel like I’m drowning most days. But when I come up and take a breath and look around, it’s quite amazing to see people working together and making innovation happen.”
The next part of the project will be the most visible. This summer, AMPF will nearly double in size as walls come down and usable space in the building is reallocated to expand the footprint to 58,000 square feet. It will be the foundation for what Stebner is most excited about.
“Right now, in manufacturing, a piece of equipment — a turbine rotor blade, for example — is created in one place, then sent somewhere else for testing,” Stebner said. “Often it goes across the country to check its interior structure, then is shipped to a second location to test its chemical composition. Georgia Tech’s plan is to put the entire process under the same roof to create a testbed for AI to perform research and development using models that it learns across the manufacturing and quality data.”
In short, Georgia Tech will make machine parts while simultaneously checking their composition, durability, and more — all made possible by AMPF’s connected machines. The devices will “talk” to each other using AI. This will ensure that engineers are making the things they think they’re making, rather than sending them around the country and waiting for confirmation. Co-locating those processes would make manufacturing more efficient and economical and provide the nation with a testbed designed for AI innovations.
“No other facility in the nation is built to do this. Georgia Tech will be the first,” Stebner said.
The construction and build-out of the new space should finish this fall. Small-scale testing of the interconnected machines will begin in 2025. Stebner’s team is about eight years away from producing large projects at scale.
“I often don’t take the time to appreciate it, because day-to-day, I feel like we’re always behind and not getting to where we need to go,” Stebner said. “But we’ve really come a long way in short time. And there’s a lot more to do.”
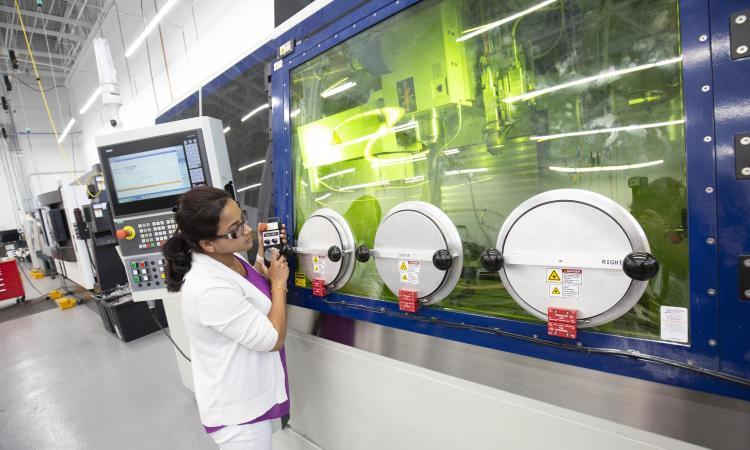
Building Georgia with AI and Manufacturing
College of Engineering faculty to lead $65 million grant announced by White House.
(text and background only visible when logged in)
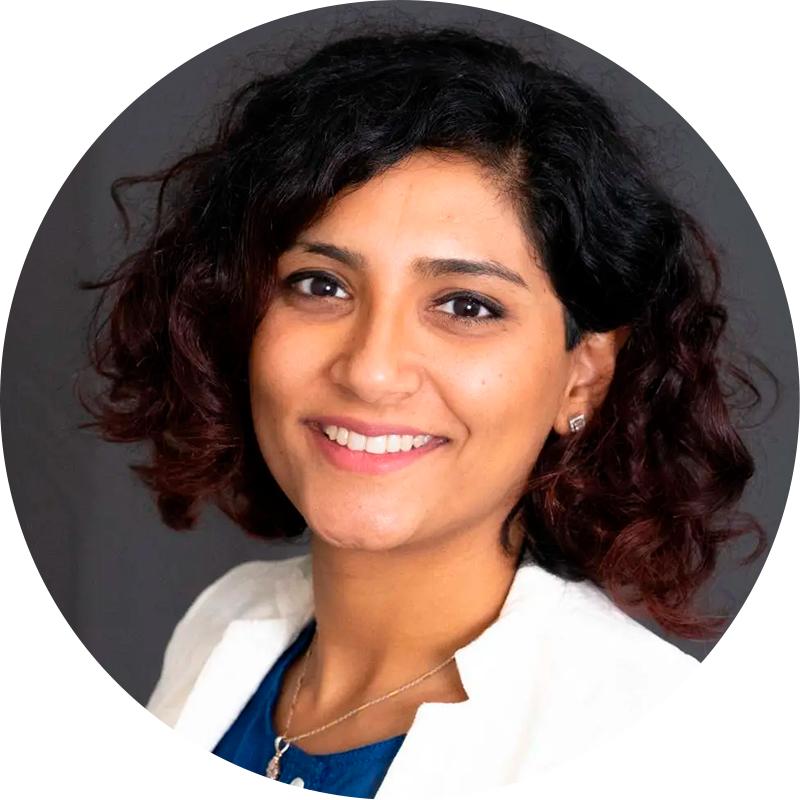
Divya Mahajan
Building Next-Gen AI Infrastructure
Divya Mahajan is focused on building the systems infrastructure and architectures we’ll need to power the AI applications and hardware emerging now and coming in the not-very-distant future. That includes breaking away from traditional systems based on central-processing units.
One current project with Ph.D. students Seonho Lee and Irene Wang is developing new AI infrastructure with energy use and efficiency as an important metric, which Mahajan said will be key to the sustainable growth of AI applications and hardware.
Mahajan is an assistant professor in the School of Electrical and Computer Engineering (ECE). She has built machine learning hardware for a decade, including time at Microsoft where she saw the real-world challenges of these large-scale systems.
“My academic position offers me the opportunity to tackle these challenges from a new perspective, enabling me to design equitable solutions that can achieve a broader impact,” she said. “I am excited to be at the forefront of the hardware and domain-specialized systems for AI, while working with students on these cutting edge and challenging problems.”
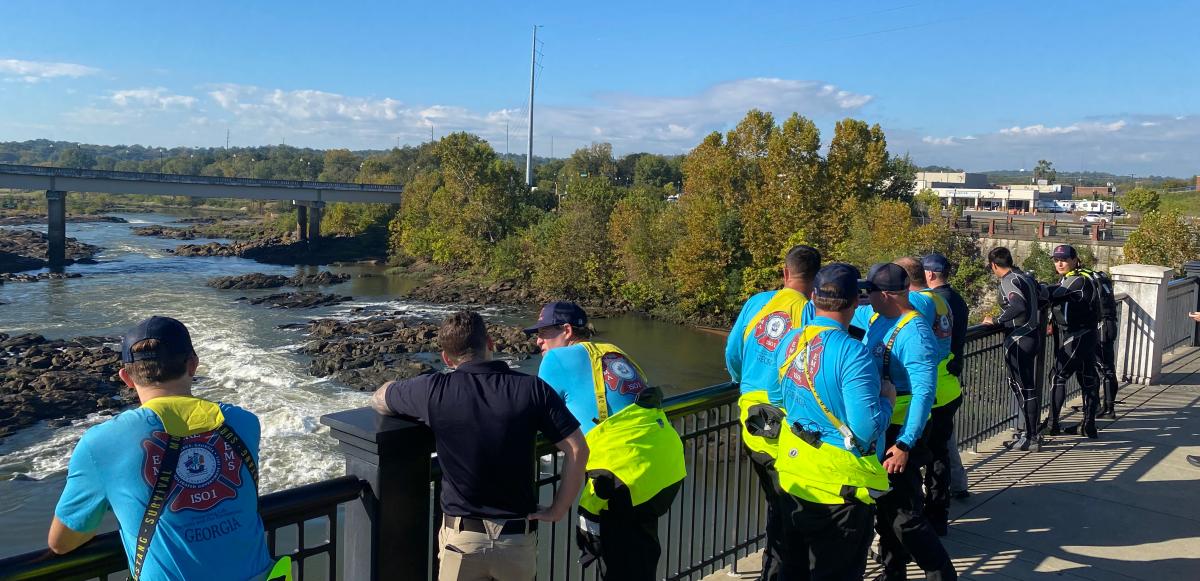
Emergency responders look over a series of rapids on the Chattahoochee River in Columbus, Georgia. (Photo: Blair Meeks)
A Safer River
The stretch of the Chattahoochee River running through downtown Columbus, Georgia, provides some of the best whitewater kayaking in the state. A riverwalk along the banks of the picturesque, twisting, turning water draws residents and visitors to walk or bike. And tiny stone islands exposed when water levels are low are almost irresistible for a little rock-hopping.
The area’s emergency responders know all too well that this ribbon of beauty presents potential dangers. When an upstream dam opens, water levels can rise in minutes. People unfamiliar with the area can quickly become trapped or swept away; there are rescues and drownings in this area of the Chattahoochee every year.
Neda Mohammadi and John Taylor in CEE have worked with Columbus to deploy a new alert system. Using cameras, a computer model of the river known as a digital twin, and AI, the system warns first responders when people are in danger.
“Research in our lab has been continually moving closer to directly impacting people’s lives. That’s what’s exciting about this project,” said Taylor, the Frederick Law Olmsted Professor. “We’re able to use these tools to actually improve safety.”
(text and background only visible when logged in)
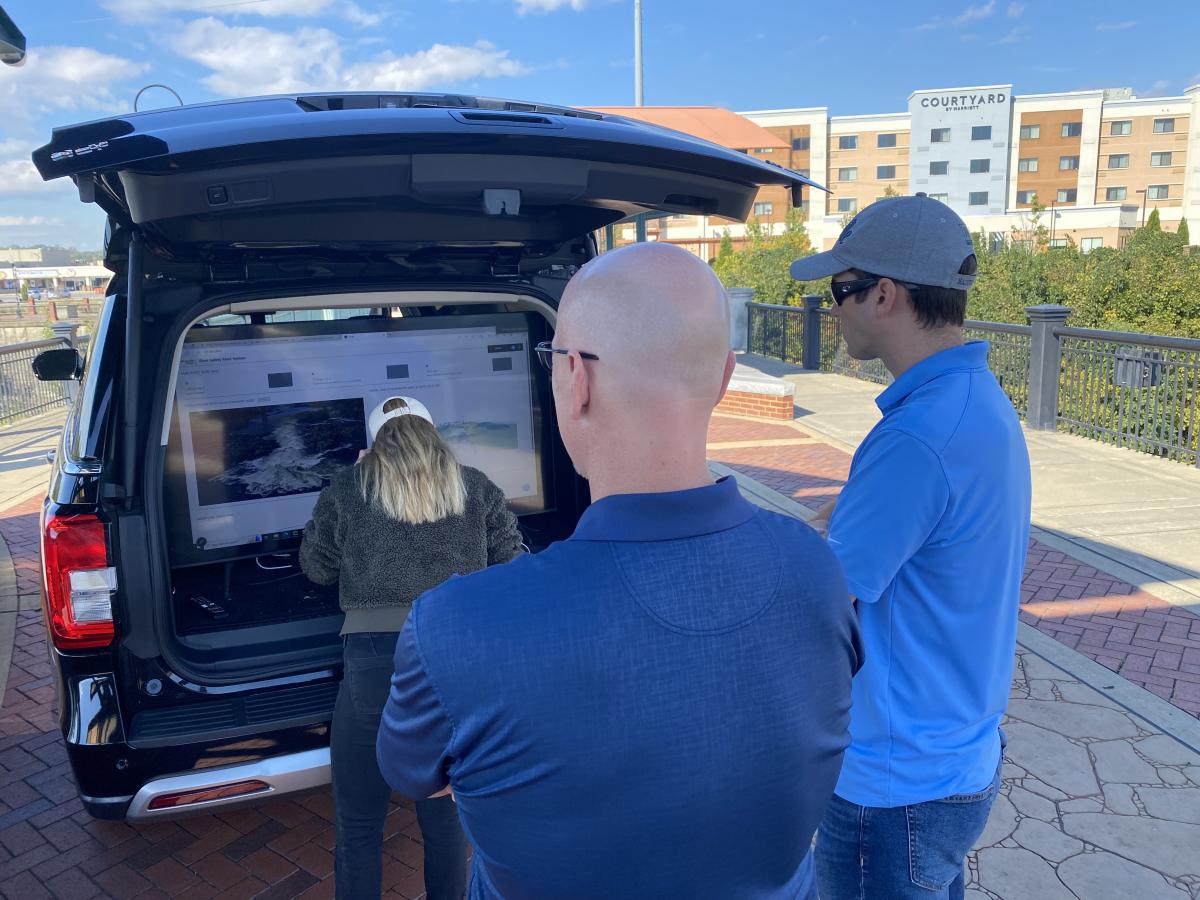
Neda Mohammadi and John Taylor monitor the Smart River Safety system during testing. (Photo: Blair Meeks)
The Smart River Safety system provides a yellow-orange-red alert based on a combination of where people are detected in the river basin and a prediction of whether water levels are going to rise. Alerts come with precise location information so emergency crews know when they’re needed and, more importantly, where.
“It means time,” said Columbus Fire and Emergency Services Captain Stephen Funk. “It means a matter of life and death. And it means having the right people in the right place.”

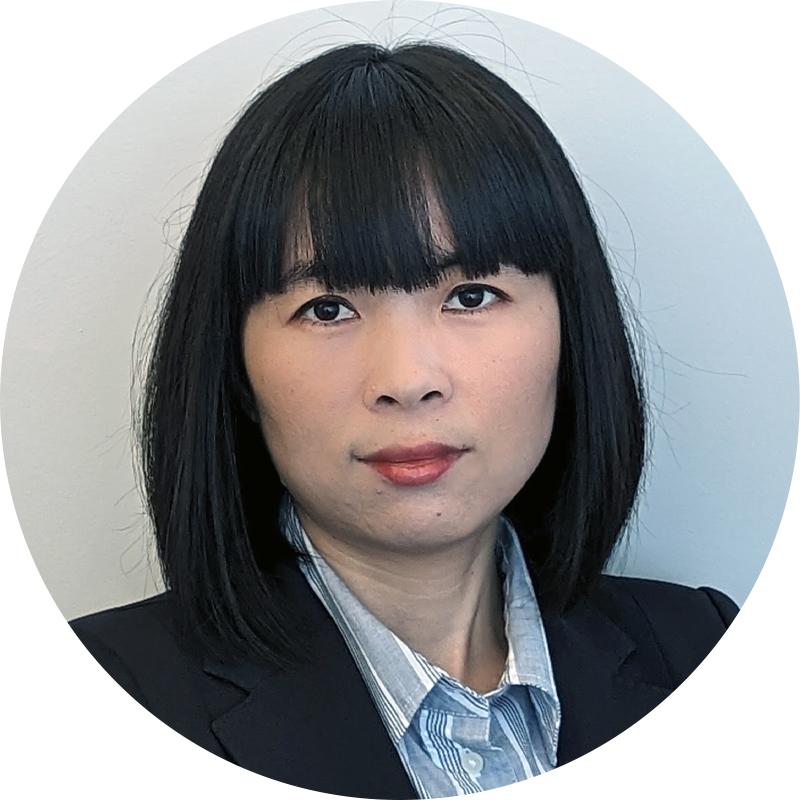
Emma Hu
High-Performance, Sustainable Fuel Cells
A promising kind of fuel cell uses platinum as a catalyst in an oxygen-reduction reaction. While the technology offers benefits — high energy density, rapid refueling, environmental friendliness — using relatively rare platinum means the fuel cells are expensive.
Emma Hu in the School of Materials Science and Engineering (MSE) is working to find other potential catalysts for these proton exchange membrane fuel cells so they don’t require platinum. Her team is particularly focused on dual-atom catalysts, using machine learning models to evaluate possible materials and offer practical guidelines for creating them in the lab.
So far, her models have analyzed the catalytic activity of more than 22,000 candidates and found roughly 3,000 that warrant further study.
“The machine learning workflow we developed allowed us to discover many more new catalyst materials than previously possible with conventional methods,” she said.
“Furthermore, our framework can be extended to other important electrochemical reactions, including carbon dioxide reduction and hydrogen evolution. We are excited about the potential of AI for addressing these challenges in sustainable energy conversion.”
Now Hu’s team is refining their models to improve its predictions. They’re also evaluating the practicality of synthesizing the dual-atom catalysts they’ve discovered and working with collaborators to demonstrate their performance experimentally.
(text and background only visible when logged in)
Creating Sensors to See and Think Through the Digital Clutter
You’re talking to your best friend in the middle of a crowded cafe. Your focus is on her, as is your gaze. But one shift of your eyes makes you aware of the scenes that surround you.
A server passes in the distance. A family studies the menu at a neighboring table. A couple leaves the bar after paying the check.
It’s all in your peripheral vision, beckoning for your attention. But your eyes and brain work together to keep you locked on your friend’s face and words. They’re able to decide what matters (her) and sift out what doesn’t (everyone and everything else).
A team of researchers, led by Saibal Mukhopadhyay, hope to replicate this same type of closed-loop system as part of a $32 million center they are leading on behalf of 12 universities. The center’s goal is to create new sensor chips that only capture and extract the most useful information from the environment, just like the human eye and brain, to sense what matters most for a given task.
It’s a substantial upgrade from today’s electronics sensors. They sample everything they “see” and generate an abundance of digital data — often way too much for the sensors to transmit and machines to store, process, and make sense of. In the process, the sensors capturing the data and the computers processing them consume an unsustainable amount of energy.
“Our center is focused on sensing to action. We’re trying to create new types of sensors that learn how to sense and absorb the most useful data. We call this cognitive sensing,” said Mukhopadhyay, the Joseph M. Pettit Professor in the School of Electrical and Computer Engineering (ECE).
The CogniSense team began the five-year project a year ago, with funding provided by the Semiconductor Research Center-administrated Joint University Microelectronics Program 2.0 (JUMP 2.0). Among the 20 faculty and 100 students working on the center are Justin Romberg, Schlumberger Professor and ECE’s associate chair for research, and Muhannad Bakir, Dan Fielder Professor and director of the Packaging Research Center. The team will demonstrate the concept of cognitive sensing for radars and lidars for applications in robots and autonomous vehicles or drones.
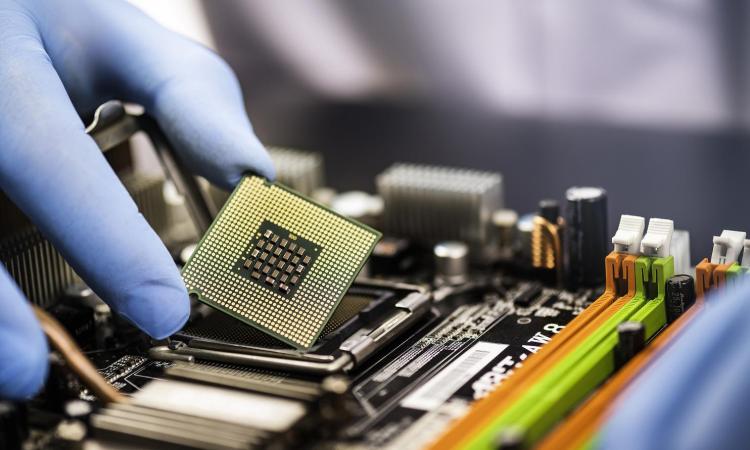
ECE Teams Receive $65 Million Grant for JUMP 2.0 Intelligent Machine Research Centers
Researchers will focus on AI cognition and intelligent sensing to action with Semiconductor Research Corporation and DARPA support.
“Cognitive sensing would be ideal in search-and-rescue missions during and after natural disasters, for example,” Mukhopadhyay said. “Radar signals can see through obstacles, such as buildings and collapsed debris. If these sensors could see, process, and learn, it would provide invaluable information to people, who could then react to what the technology discovers.”
The first year of the center primarily pulled the multi-university group together, learning what each can contribute to the overall effort. The team also built prototypes and showed how artificial intelligence and signal processing methods can make judgments about what information should and should not be encoded.
The next step is connecting those discoveries across the team’s disciplines to someday create the sensors and expand access to semiconductor research.
“Through the exploration of cognitive multi-spectral sensors, the CogniSense Center ignites a passion for innovation and research, illuminating the path for a new generation of students to discover the endless possibilities within our center,” said Devon McLaurin, senior program and operations manager. “Together, we strive to foster curiosity, nurture creativity, and empower students to become the pioneers of tomorrow’s breakthroughs.”
The CogniSense initiative is one of two JUMP 2.0 centers in ECE. The other $32 million center is headed by Arijit Raychowdhury, the Steve W. Chaddick Chair. It focuses on AI systems that continuously learn from human interactions to enable better collaboration between people and AI and ultimately build a digital human.
(text and background only visible when logged in)
(text and background only visible when logged in)
Pushing the Edge of Mobility
Robotic exoskeletons that could protect workers from injuries or help stroke patients regain their mobility so far have been largely limited to research settings. Most robotic assistance devices have required extensive calibration for each user and context-specific tuning.
Aaron Young’s mechanical engineering lab is on the verge of changing that with an AI-driven brain for exoskeletons that requires no training, no calibration, and no algorithm adjustments. Users can don the “exo” and go. Their universal controller works seamlessly to support walking, standing, and climbing stairs or ramps. It’s the first real bridge to taking exoskeletons from research endeavor to real-world use.
The secret to Young’s controller is a complete change in what the algorithms are trying to do. Instead of focusing on understanding the environment and predicting how to help the wearer do whatever they’re doing, this controller focuses on the body.
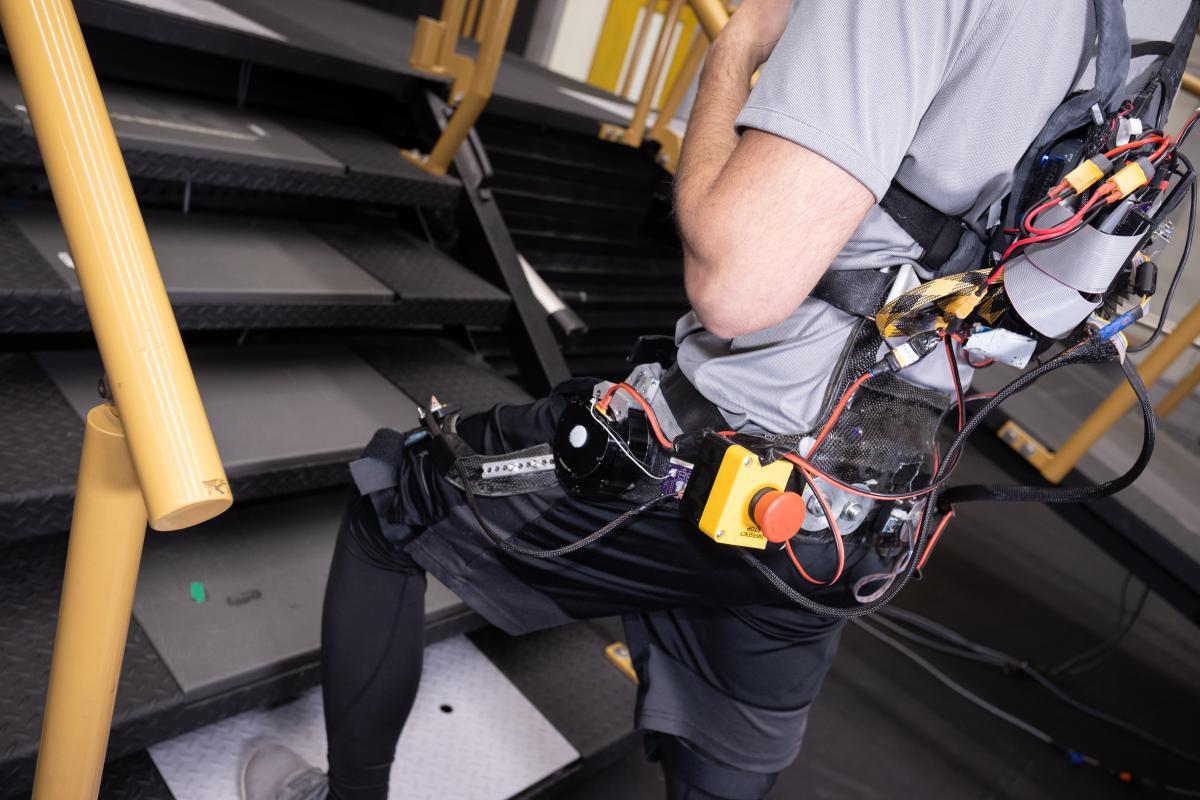
The exoskeleton and Young’s universal controller work seamlessly to support activities like climbing stairs with no calibration. (Photo: Candler Hobbs)
“The idea is to take all the cues from the human,” Young said. “What were the human joint torques? What were the moments that their muscles were generating as they did these different activities? Our controller is simple and elegant: It basically delivers a percentage of the user’s effort.”
Young’s lab also is using AI to help amputees use robotic prostheses to more easily navigate the world and help older adults, or those with mobility issues, maintain their balance.
(text and background only visible when logged in)
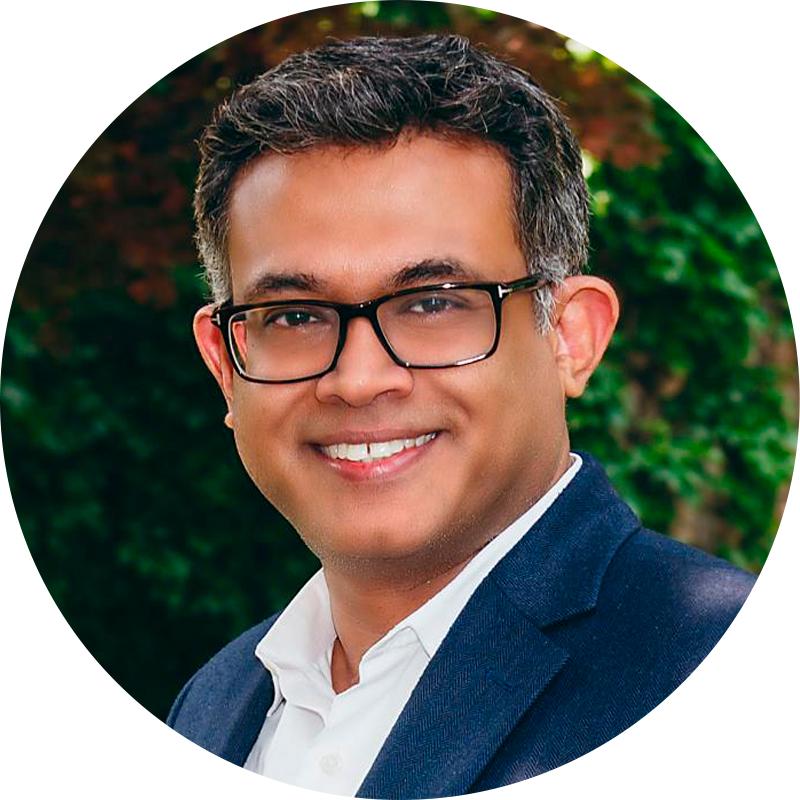
Suman Datta
More Compute Power, More Efficiently
A team of electrical and computer engineers recently secured $9.1 million from the Defense Advanced Research Projects Agency (DARPA) to help advance AI hardware.
The project will develop new compute-in-memory accelerator technology, which aims to greatly increase the energy efficiency and computational throughput of devices used in AI-based applications like image analysis and classification. It’s led by Suman Datta, Joseph M. Pettit Chair in Advanced Computing and professor in ECE and MSE.
Their team’s approach turns typical computer architecture on its head. Instead of moving data back and forth from memory to a central processing unit for computation, the researchers are developing compute-in-memory hardware designs to minimize data movement and conserve energy. The keys to their work are what are called multiply accumulate (MAC) macros.
“In the context of AI inference, MAC operations are crucial for performing computations efficiently in neural networks,” Datta said. “The ability to efficiently execute MAC operations is essential for optimizing the performance of AI models on various hardware platforms like CPUs, GPUs, and custom AI chips like the one we are developing for DARPA.”
Datta and ECE collaborators Saibal Mukhopadhyay, Shimeng Yu, and Arijit Raychowdhury have set out to design their chips to maximize power efficiency while producing new levels of computation and minimizing size. Their goal is to build accelerators that can achieve 300 trillion operations per second per watt of power — a full order of magnitude higher than current state-of-the-art systems that might achieve 10s of trillions of operations per second.
(text and background only visible when logged in)
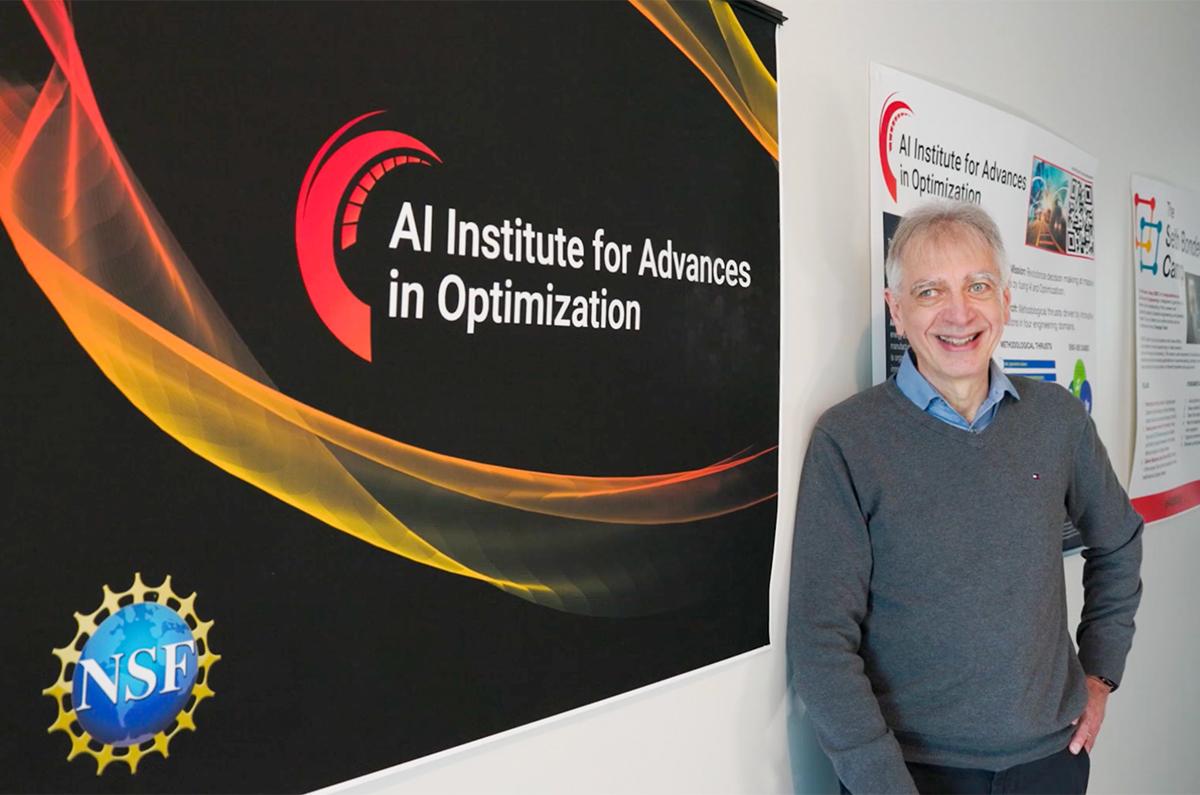
AI4OPT Director Pascal Van Hentenryck (Courtesy: AI4OPT)
AI-Driven Optimization
Established in 2021 with $20 million from the National Science Foundation (NSF), the AI Institute for Advances in Optimization (AI4OPT) is a hub of innovation at the intersection of AI and optimization.
AI4OPT Director Pascal Van Hentenryck said the effort has pushed the boundaries of research and simultaneously invested in educational initiatives and trustworthy AI.
“AI4OPT exemplifies our commitment to fusing AI and optimization to address real-world challenges,” said Van Hentenryck, who also is the A. Russell Chandler III Chair and Professor in the H. Milton Stewart School of Industrial and Systems Engineering. “Our focus on trustworthy AI ensures that our solutions are not only effective but also reliable.”
In addition to research, AI4OPT is investing in collaboration and outreach.
The Seth Bonder Camp is named for the late pioneer in computational and data science and plays a pivotal role in introducing high school students to career opportunities in industrial engineering and operations research.
Offered online and on campus at both Georgia Tech and Kids Teach Tech, AI4OPT’s educational partner in California, the camp provides students with hands-on experience and a deeper understanding of the applications of AI and optimization in engineering.
Meanwhile, the Faculty Training Program is empowering educators from historically Black colleges and universities and minority-serving institutions to integrate AI and optimization concepts into their curricula. Over three years, participants receive training in technical courses such as data mining, statistics, and machine learning, as well as course design to establish AI and optimization minors or majors at their institutions.
“After seeing much success of the first cohort, we are bringing in another cohort, marking a significant milestone in our commitment to diversity and inclusion in STEM education,” Van Hentenryck said.
AI4OPT also collaborates with industry partners to support its research through large-scale case studies. Partners provide internships for its students, too, offering real-world experience applying AI and optimization techniques.
“AI4OPT remains committed to advancing research and education in AI-driven optimization,” Van Hentenryck said. “Through initiatives like the Seth Bonder Camp and the Faculty Training Program, we aim to inspire the next generation of AI experts and promote diversity and inclusion in STEM fields.”
‣ BREON MARTIN
(text and background only visible when logged in)
(text and background only visible when logged in)
Better Decisions Faster
When engineers are creating the designs and systems that make our world function — and especially when they’re developing cutting-edge new designs — the complexity of the task is enormous. Aerospace engineer Elizabeth Qian focuses on using machine learning to create “surrogate models,” which are approximations of more complex (and more expensive) engineering simulation methods. The idea is to give engineers the ability to explore many different designs in far less time.
A key component of using AI tools to design engineering systems is trust: unlike image generation or chatbots, these tools create designs with consequences for human safety. Qian and collaborators have been working on methods to train AI models with a variety of data — some accurate but expensive to obtain, some easier or cheaper to get but less accurate — so that data requirements are manageable and the models are guaranteed to be accurate.
“There are so many critical open challenges in developing AI tools that we can trust when we use them for engineering design, and solutions to these challenges truly require interdisciplinary collaboration that unites knowledge in engineering, AI algorithms, and fundamental mathematics and statistics,” Qian said. “It’s very exciting to collaborate with colleagues to advance solutions that can make an impact in this area.”
(text and background only visible when logged in)
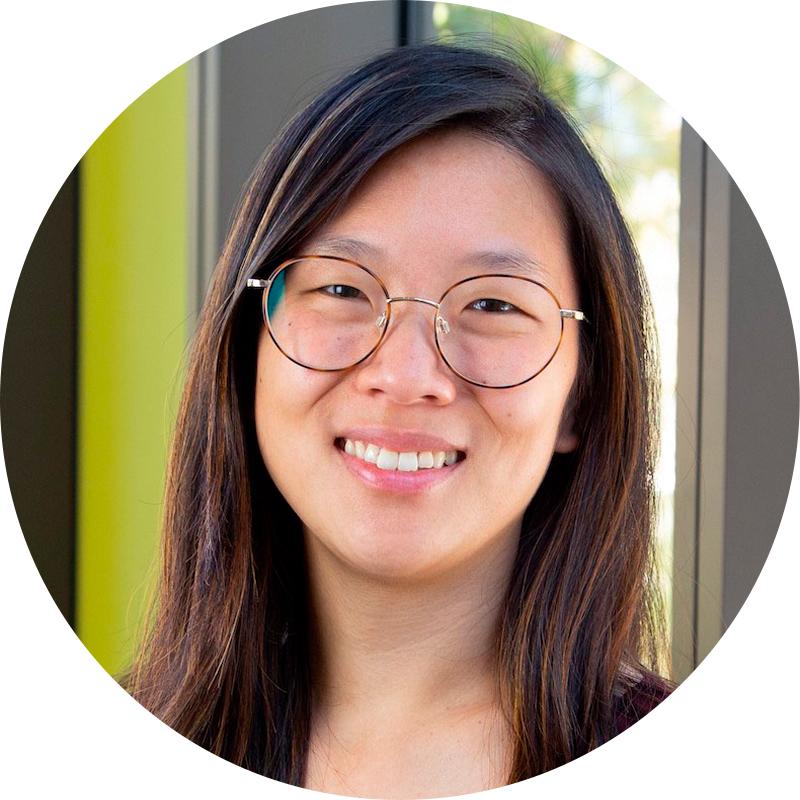
Elizabeth Qian

(text and background only visible when logged in)
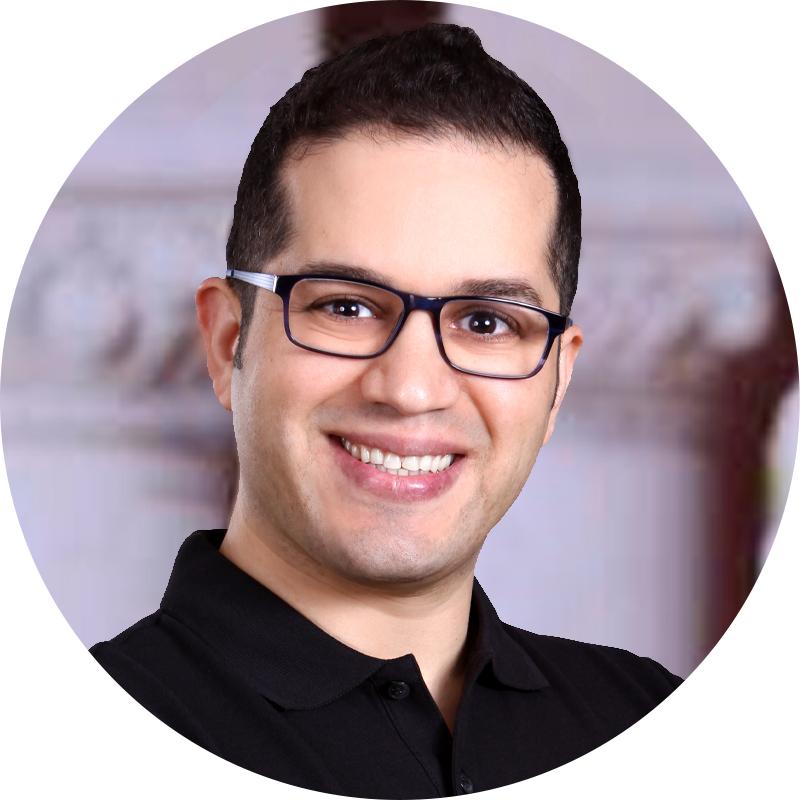
Vahid Serpooshan
Equitable Tissue Manufacturing
Working with policy scholars and mathematicians, engineers are using AI to make advanced tissue engineering more effective for patients of every background. They’re focused on a kind of 3D printing that uses commercially available stem cells as a bio-ink to create patient-specific tissue — cardiac muscle, in this case. The team will measure how those tissues function, then feed the data into an AI platform to optimize the bioprinting processes for patients of various racial and ethnic backgrounds.
“AI-enabled biomanufacturing needs large datasets,” said biomedical engineer Vahid Serpooshan. “But a great majority of studies are based on data, stem cells, and other biological materials from a very narrow population group — mainly, white males — which doesn’t accurately represent the rich diversity of humanity.”
That means even while new biotechnologies offer the promise of incredible advances, they’re also exacerbating existing health disparities. The team, which includes Emory University researchers, says the work is a first step toward training an AI model that eventually would allow efficient manufacturing of functional tissues for a wide range of patients.
(text and background only visible when logged in)
Related Stories
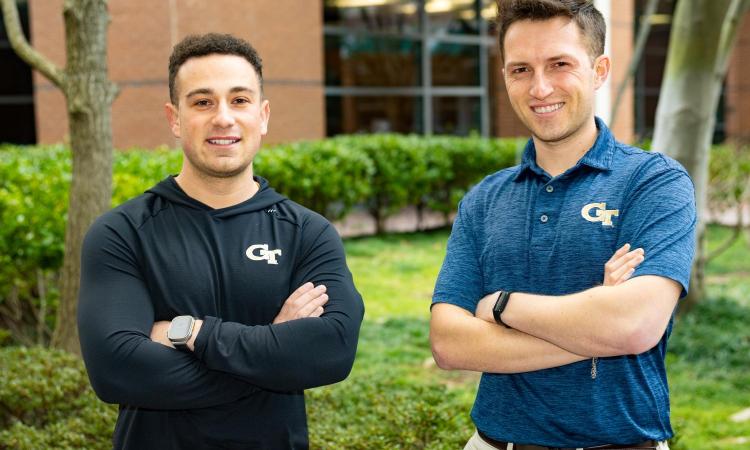
Managing the Ups and Downs
With GlucoSense, alumni are creating a single tool to help diabetes patients wrangle data to better manage their health.
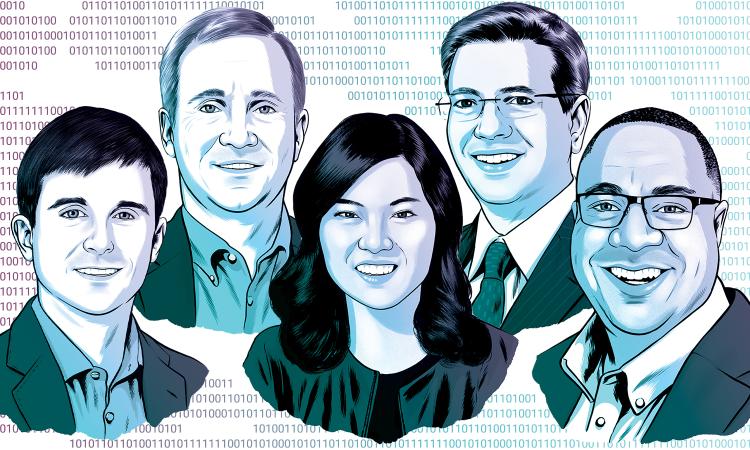
AI Beyond Campus
Corporate leaders with ties to the College describe AI in their current roles, what will happen in the next five years, and how students and professionals will need to adapt.
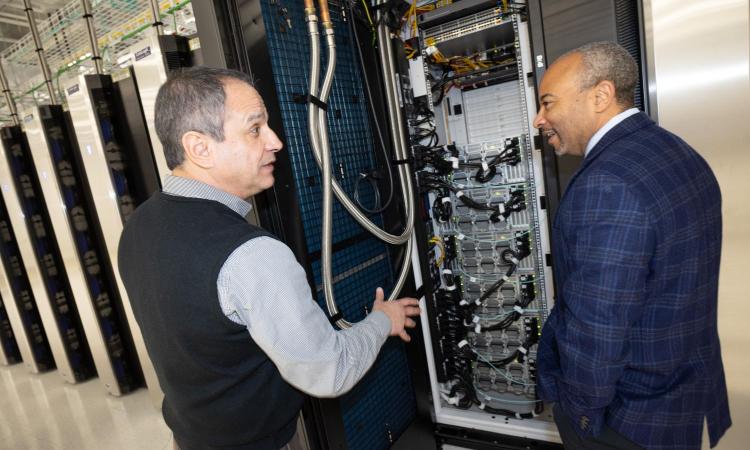
Making AI
A first-of-its-kind AI Makerspace created in collaboration with NVIDIA will give undergrads unprecedented access to supercomputing power for courses, projects, and their own innovations.
(text and background only visible when logged in)
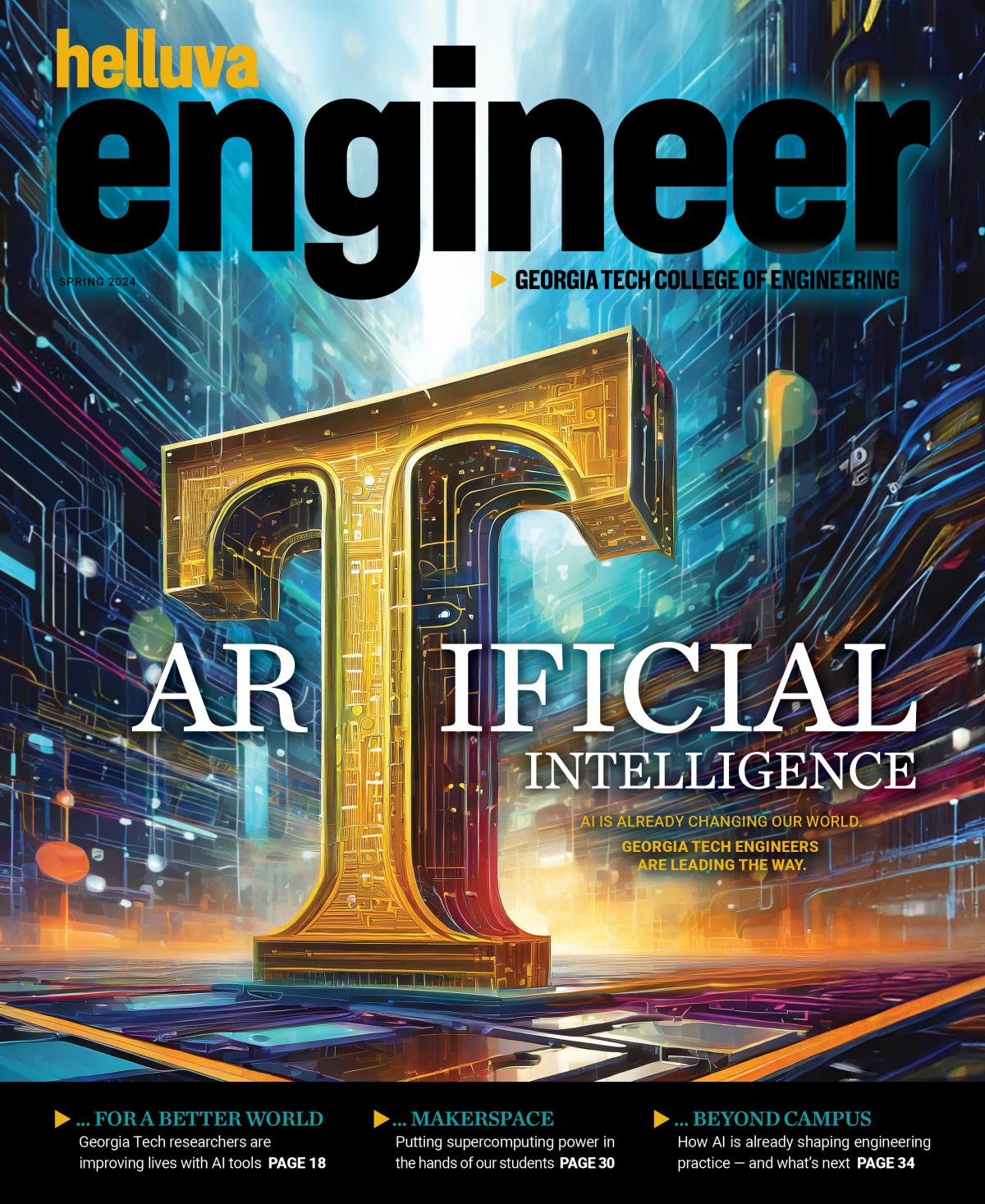
Helluva Engineer
This story originally appeared in the Spring 2024 issue of Helluva Engineer magazine.
Georgia Tech engineers are using artificial intelligence to make roads and rivers safer, restore or boost human function, and enhance the practice of engineering. We’re building the technology and infrastructure to power tomorrow’s AI tools. And we’re giving our students the AI courses and supercomputing power they need to be ready. AI is changing our world, and Georgia Tech engineers are leading the way.